Utilizing Big Data and Analytics
Leverage big data and analytics to optimize your supply chain operations, improve decision-making, and maintain a competitive edge.
SUPPLY CHAIN
The Procure 4 Marketing Team
5/15/20245 min read
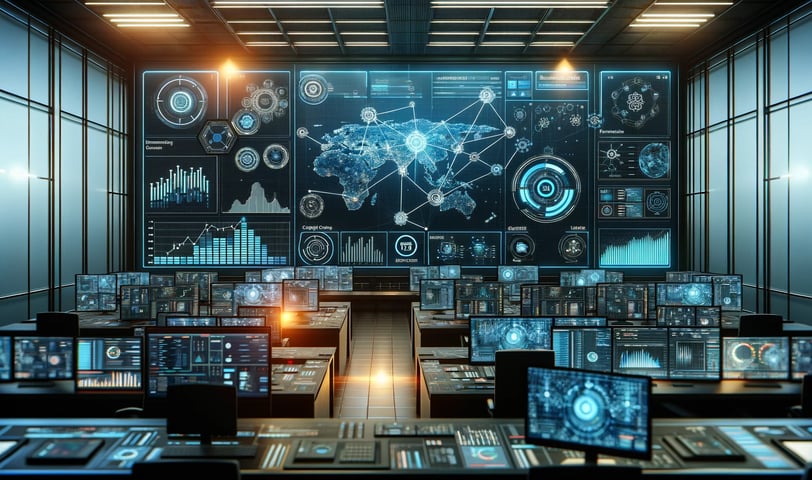
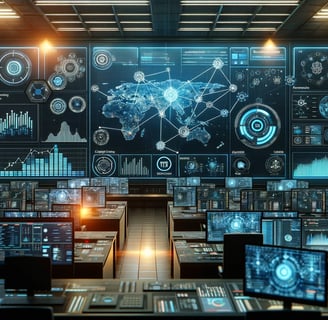
Big data and analytics refer to the vast volumes of data generated from various sources within the supply chain, combined with the advanced analytical techniques used to interpret this data. This powerful combination allows businesses to extract meaningful insights that drive smarter, more informed decision-making across the entire supply chain. In today's hyper-connected world, leveraging big data has become critical for companies aiming to optimize operations, improve customer satisfaction, and maintain competitive advantage.
The Evolution of Data Analytics in Supply Chains
Data analytics in supply chain management has evolved significantly over the past few decades. Initially focused on basic descriptive analytics to understand past performance, it has now expanded to include predictive analytics for forecasting future trends and prescriptive analytics to determine the best courses of action. The integration of IoT devices, RFID technology, and other digital tools has further enriched data collection, providing businesses with real-time insights into every aspect of their operations, from inventory levels to transportation efficiency. This evolution has transformed traditional supply chains into dynamic, adaptive systems capable of responding swiftly to changing market conditions and customer needs.
Key Benefits of Big Data in Supply Chains
Improved Forecasting and Demand Planning
Big data and analytics have significantly enhanced the ability of supply chains to forecast demand and plan inventory more effectively. By analyzing vast amounts of data from various sources, including historical sales data, market trends, consumer behavior, and even social media sentiments, analytics enable businesses to predict future demand with greater accuracy. This predictive capability allows companies to adjust their production schedules, inventory levels, and staffing requirements proactively, reducing the likelihood of overstock or stockouts and ensuring they can meet customer demands efficiently.
Enhanced Operational Efficiency
Utilizing big data in supply chain operations translates to heightened operational efficiency. Real-time data collection and analysis through sensors and IoT devices provide continuous insight into logistics operations, helping identify bottlenecks and inefficiencies promptly. This information enables businesses to optimize routes, improve transportation schedules, streamline warehouse operations, and enhance overall workflow. Consequently, this leads to reduced operational costs, quicker delivery times, and improved service quality, directly impacting the bottom line and customer satisfaction.
Risk Management
Big data plays a crucial role in risk management within supply chains. By aggregating and analyzing data across the entire network, businesses can identify potential risks much earlier than traditional methods would allow. This includes detecting vulnerabilities in the supply chain, forecasting disruptions like supply shortages or delivery delays, and even monitoring for signs of fraud or cyber threats. Armed with this information, companies can develop robust strategies to mitigate these risks, such as diversifying suppliers, adjusting inventory strategies, or reinforcing cybersecurity measures.
In sum, big data analytics offers transformative benefits for supply chains, improving forecasting and demand planning, boosting operational efficiency, and enhancing risk management. These advantages make big data not just a technological asset but a strategic imperative for modern supply chains aiming to thrive in an increasingly complex and volatile business environment.
Technological Foundations of Big Data in Supply Chains
Data Collection Technologies
Big data in supply chains is primarily sourced through a variety of advanced technologies that collect detailed information across all stages of the supply chain:
IoT Devices: Internet of Things (IoT) devices are embedded in various supply chain elements, from manufacturing equipment to transportation vehicles, continuously transmitting data about operational status, environmental conditions, and more.
RFID Tags: Radio Frequency Identification (RFID) tags are used extensively for tracking inventory as it moves through the supply chain, providing real-time data on the location and status of products.
Online Platforms: E-commerce platforms and online transaction systems also contribute significantly to big data, offering insights into consumer purchasing behaviors and preferences.
Analytical Tools and Techniques
To transform this vast amount of data into actionable insights, several analytical tools and techniques are employed:
Statistical Methods: Basic and advanced statistical analyses help identify trends and patterns, enabling predictive analytics.
Machine Learning Algorithms: These algorithms learn from historical data to improve their predictions over time, making them particularly useful for demand forecasting and anomaly detection in supply chain operations.
Visualization Tools: Data visualization tools and dashboards convert complex data sets into understandable and actionable visuals, helping managers make informed decisions quickly and effectively.
Together, these technologies and tools form the backbone of big data analytics in supply chains, enabling businesses to optimize operations and enhance strategic decision-making.
Challenges in Implementing Big Data Analytics in Supply Chains
Data Quality and Quantity
One of the primary challenges in leveraging big data within supply chains is ensuring both the quality and quantity of the data collected. Accurate, clean, and comprehensive data is crucial for effective analysis and decision-making. However, data may often be incomplete, incorrect, or inconsistent, especially when gathered from disparate sources across various stages of the supply chain. Additionally, the sheer volume of data can be overwhelming, and without proper data management strategies, it can lead to analysis paralysis where collecting and sorting data overshadows actionable insights.
Integration with Existing Systems
Integrating big data analytics into existing supply chain systems poses significant technical and operational challenges. Many organizations operate on legacy systems that are not initially designed to handle the scale and complexity of big data analytics. Upgrading these systems or developing new ones that can seamlessly interact with modern big data solutions requires substantial investment in technology and changes to existing workflows. Ensuring compatibility and maintaining continuous operations during this transition can be complex and resource intensive.
Skill Gaps
The effective implementation of big data analytics also demands a specific skill set that includes data science expertise, understanding of machine learning, and proficiency in statistical analysis. These skills are not traditionally associated with supply chain management roles. As a result, there is often a significant skill gap in organizations, making it challenging to harness the full potential of big data analytics. Investing in training for current employees or recruiting new talent with the necessary technical expertise is essential for overcoming these skill gaps and successfully applying big data insights to improve supply chain operations.
Navigating the challenges of data quality and quantity, system integration, and skill gaps is crucial for organizations looking to implement big data analytics in supply chains. Addressing these issues not only enhances operational efficiency but also drives innovation, providing a competitive edge in the rapidly evolving market landscape.
Future Trends in Big Data and Analytics
As big data continues to redefine the landscape of supply chain management, the integration of advanced technologies like artificial intelligence (AI) and machine learning (ML) is set to further enhance the power of predictive analytics. These technologies enable more accurate forecasting and smarter decision-making by learning from data patterns and improving over time. This evolution points towards a future where supply chain responses are not only reactive but also proactive, anticipating disruptions and demands before they occur.
Additionally, augmented reality (AR) and virtual reality (VR) are emerging as powerful tools for data visualization and decision-making in supply chains. By overlaying data visualizations onto the real world or simulating supply chain operations in a controlled virtual environment, these technologies provide an immersive insight into complex datasets and operational workflows, enhancing understanding and strategic planning.
The transformative potential of big data and analytics in revolutionizing supply chain management cannot be overstated. As we move into a data-driven world, the ability to efficiently analyze large datasets and extract actionable insights is becoming increasingly critical. Businesses are encouraged to invest in developing or enhancing their data analytics capabilities, ensuring they remain competitive and well-equipped to navigate the complexities of modern supply chains.
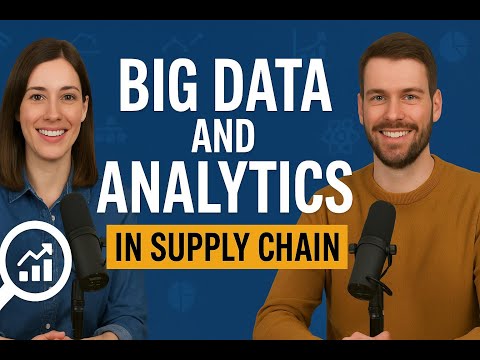