Data Analytics in Demand Forecasting
Explore how data analytics revolutionizes demand forecasting in supply chains, enhancing accuracy and efficiency with advanced technologies.
SUPPLY CHAIN
The Procure 4 Marketing Team
6/5/20245 min read
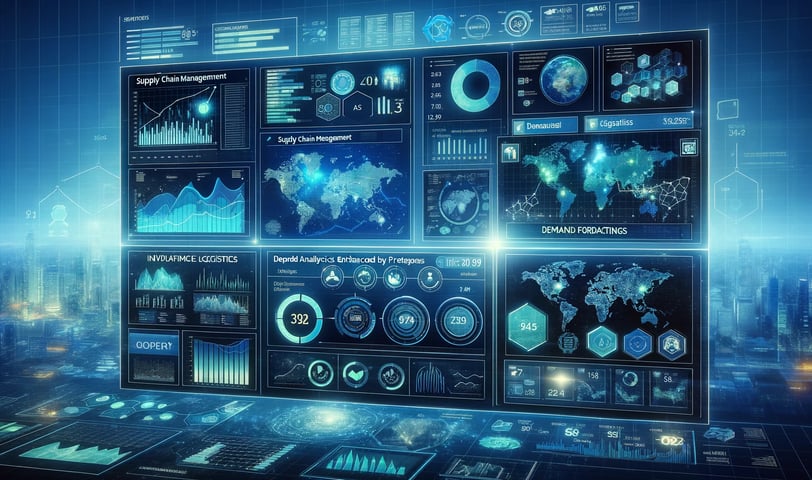
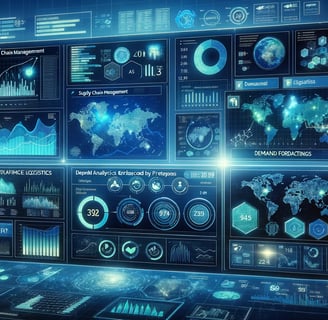
Data analytics encompasses the techniques and processes of analyzing raw data to extract valuable insights and information. In the context of demand forecasting within supply chain management, data analytics is indispensable for deciphering complex patterns and predicting future demand. This predictive capability allows companies to align their production schedules, inventory levels, and distribution strategies with anticipated market demands, thereby enhancing efficiency and reducing costs.
The evolution of data analytics has been pivotal in transforming supply chain operations. Initially, businesses relied on simple statistical tools and historical sales data to estimate future needs. However, as technology advanced, so did the analytics, evolving into sophisticated systems capable of integrating and interpreting vast arrays of data from varied sources. Today, advanced data analytics utilize everything from machine learning algorithms to real-time data streams, enabling a more dynamic and responsive approach to forecasting.
This progression has made data analytics an integral part of strategic planning in supply chains, providing decision-makers with the insights needed to make informed, proactive choices that keep their operations competitive and aligned with market conditions.
Role of Data Analytics in Demand Forecasting
Predictive Analytics
At the heart of demand forecasting within modern supply chains is predictive analytics, a branch of data analytics that uses historical data and statistical algorithms to forecast future outcomes. By analyzing past sales trends, seasonal variations, and other relevant historical data, predictive analytics models can identify patterns and predict future demand with a high degree of accuracy. These models, often powered by machine learning, continuously improve as they process new data, becoming more precise in their predictions over time. This allows companies to anticipate market needs, optimize inventory, and plan production more effectively.
Real-Time Data Utilization
The importance of real-time data in demand forecasting cannot be overstated. Real-time data analytics enable businesses to monitor ongoing conditions and instantly adjust their forecasts and operations accordingly. This responsiveness is crucial for adapting to sudden market changes such as unexpected shifts in consumer demand or supply chain disruptions. By integrating real-time data from point-of-sale systems, online transactions, and IoT devices, companies can maintain a high level of agility, ensuring that their supply chain operations are always aligned with current market conditions.
Integration of External Factors
Beyond internal data, effective demand forecasting also involves the integration of external variables. Data analytics can incorporate a wide range of external factors, including market trends, economic indicators, competitive actions, and consumer behavior insights. For example, changes in economic conditions or consumer preferences can dramatically influence demand patterns. By analyzing these external data sources, predictive models can adjust forecasts to better reflect the broader economic and social landscape. This holistic approach ensures that demand planning is not only based on internal company data but is also responsive to the outside world, leading to more robust and adaptable supply chain strategies.
By leveraging predictive analytics, utilizing real-time data, and integrating external factors, data analytics provides a comprehensive toolset for enhancing demand forecasting in supply chains, ultimately leading to more informed decision-making and optimized operations.
Technological Foundations of Data Analytics in Demand Forecasting
Big Data Technologies
The backbone of modern data analytics in demand forecasting is big data technologies. These technologies encompass a variety of tools and platforms designed to handle vast amounts of data that are too large and complex for traditional data-processing software. Big data platforms, such as Hadoop and Spark, allow for the efficient processing and analysis of huge datasets by distributing the data across many servers. Additionally, cloud computing plays a crucial role by providing scalable and flexible resources for storing and analyzing data. Cloud services enable companies to access immense computational power on-demand, without the need for substantial upfront investment in physical hardware, making advanced data analytics accessible to a wider range of businesses.
Machine Learning Techniques
In demand forecasting, specific machine learning techniques are utilized to enhance predictive accuracy. Time series analysis is a prevalent method, focusing on sequential data points collected over time to forecast future values. This technique is particularly suited for analyzing sales trends and seasonal effects. Regression models, another common approach, predict a dependent variable based on one or more independent variables, providing valuable insights into how different factors might influence demand. These machine learning models are integral to processing and making sense of the data collected, allowing businesses to extract actionable insights and make data-driven decisions efficiently.
Together, big data technologies and machine learning techniques form the technological foundation of data analytics in demand forecasting, enabling businesses to harness the full potential of their data for more accurate and dynamic supply chain management.
Challenges and Considerations in Implementing Data Analytics for Demand Forecasting
Data Quality and Management
One of the foremost challenges in utilizing data analytics for demand forecasting is ensuring the quality and management of the data. Accurate, clean, and consistent data is crucial for effective analysis; however, data often comes from various sources and can include inaccuracies, inconsistencies, or redundancies. Companies must invest in robust data management systems and processes that standardize data collection, clean, and validate data inputs, and reconcile data from different sources. This effort ensures that the data used for analysis is reliable and that the forecasts generated are valid, helping to prevent costly errors in decision-making.
Integrating and Updating Technology Systems
Integrating new data analytics tools with existing IT infrastructure presents significant technical challenges. Many organizations operate on legacy systems that might not be compatible with the latest analytics technologies. Updating these systems can be costly and disruptive to ongoing operations. Additionally, integration must be handled delicately to maintain data integrity and system security. Organizations need to plan these integrations carefully, often requiring phased rollouts or parallel runs to ensure that the new systems function as intended without affecting the overall business processes.
Skills and Expertise
The effective use of data analytics in demand forecasting also demands a specific set of skills and expertise. There is a critical need for skilled data analysts, data scientists, and machine learning experts who can not only manage and analyze large datasets but also interpret the results to derive actionable business insights. The talent pool for such expertise is competitive, and organizations may struggle to attract and retain qualified professionals. Providing training and continuous learning opportunities for existing staff can be a viable alternative to help bridge this skills gap.
Navigating these challenges is essential for organizations looking to leverage data analytics effectively in their demand forecasting efforts. Addressing issues related to data quality, technological integration, and workforce expertise is crucial in maximizing the benefits of data analytics, ultimately leading to more informed and strategic decision-making in supply chain management.
Future Trends in Data Analytics for Demand Forecasting
As we look to the future, data analytics in demand forecasting is poised for significant advancements through emerging technologies and methodologies. Innovations such as artificial intelligence and machine learning continue to evolve, offering even more sophisticated predictive capabilities. These technologies are increasingly integrated with real-time data streams from IoT devices, enhancing the accuracy and timeliness of forecasts. Additionally, advancements in cloud computing are enabling more scalable analytics solutions, allowing businesses of all sizes to leverage powerful predictive tools without substantial upfront investment.
The integration of augmented reality and virtual reality technologies is also on the horizon, poised to transform how data is visualized and interacted with, making complex data sets more accessible and understandable for decision-makers. These trends indicate a future where demand forecasting is not only more accurate but also more integrated with daily operations, providing a seamless flow of actionable insights.
In conclusion, the impact of data analytics on enhancing demand forecasting accuracy and operational efficiency cannot be overstated. Businesses are encouraged to embrace these advanced analytical tools to stay competitive in a rapidly evolving market. Adopting these technologies not only improves operational capabilities but also positions companies to be more agile and responsive to market dynamics, ensuring they remain at the forefront of innovation in supply chain management.